Virtual reality (VR) has been studied for decades, but only recently has it reached the kind of mainstream visibility that fuels intensive R&D. In this thesis, Stefan Pelser—under the supervision of Dr Rensu Theart—investigated how convolutional neural networks (CNNs) can translate real-world objects into the virtual domain.
The core objective of this research being to deliver a VR experience in which sight and touch finally agree. In addition, CNN-driven object mapping promises to bridge that gap, letting users see the very items they are holding and, therefore, feel their true texture, weight, and resistance.
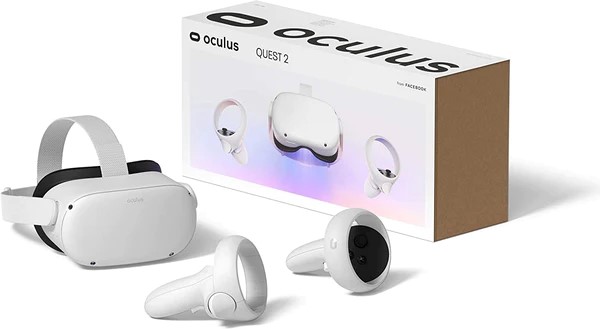
Figure 1: Meta Quest 2.
Methodology, Datasets, and Pose-Estimation Pipeline
The project started with a suite of synthetically generated datasets that followed the LineMOD format, augmented to cover varied lighting, backgrounds, and occlusions. These datasets fed EfficientPose, a state-of-the-art 6DoF pose-estimation network. Then, real-world capture occurred via the headset’s own spatial-mapping sensors, while two Logitech C270 webcams supplied RGB data to EfficientPose in real time.
To benchmark the deep-learning approach, an ArUco-marker pipeline was implemented in parallel. Both pipelines relayed their pose outputs to the VR engine, which rendered each tracked object in the user’s field of view with minimal latency.
Tactile Immersion—Why Mapping Matters
Despite efforts to make VR as close to reality as possible, there remains a significant gap. The current technology does not allow users to physically feel the virtual environment, which impacts the immersion of the VR experience. But visual fidelity alone cannot sustain presence if your hands never “belonged” in the scene.
That’s why plastic controllers remind you of their shape; controller-free hand tracking offers no haptic cues. Mapping the actual mug, wrench, or surgical tool you hold into the headset view restores the missing tactile channel, delivering:
- Texture and grain—feel the wood of a hammer handle or the ridges of a game prop.
- Weight and inertia—lift a real dumb-bell and see its twin move identically in VR.
- Temperature cues—sense a cold metal scalpel during medical simulation.
Because this approach uses inexpensive cameras and household objects, it avoids the cost and complexity of specialized haptic gloves or bespoke peripherals.
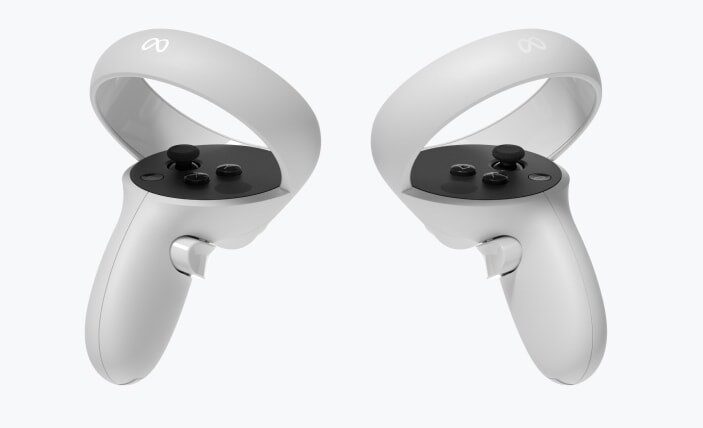
Figure 2: Meta Quest 2 controllers and breakdown of Quest controllers’ inputs
Experimental Objects and Evaluation
Four items test the system’s breadth:
- LineMOD duck (baseline, asymmetric)
- Cube (symmetric) – both textured and textureless
- Knife (elongated, reflective)
- Handgun prop (complex silhouette)
Two integration pipelines were compared: meshes reconstructed via photogrammetry vs. CAD files from 3-D-printing repositories. The performance metrics include frame-to-frame jitter, absolute pose error, and recovery time after occlusion.
Results and Observations
EfficientPose demonstrates strong performance with sub-centimetre accuracy on asymmetric objects like the duck and handgun, proving more robust than ArUco markers when those markers are obscured. A challenge arises with highly symmetric objects, exemplified by the cube, where accuracy drops.
This suggests that standard 6DoF networks struggle with rotational ambiguity unless training data is designed to compensate. To improve reliability, texture plays a role, but even without it, tailored data augmentation allows the textureless cube to be tracked within ±1.8 cm.
Another limitation is user-induced occlusion; covering more than 40% of the object with a hand can temporarily lead to pose loss. Fortunately, multi-camera triangulation offers a viable solution to this occlusion problem and indicates a scalable approach for future development.
Deep-Learning Hurdles on the Path to Low-Cost Adoption
From the research, three challenges surfaced as priorities for future work:
- Model suitability – Lightweight architectures or pruning may be required to hit real-time targets on entry-level GPUs while retaining robustness against symmetry and glossy surfaces.
- Data acquisition – Synthetic augmentation accelerates dataset growth, yet biases from lighting and background variance must be managed to prevent brittle performance in new environments.
- Operational resilience – The system must withstand clutter, motion blur, and rapidly changing shadows found in classrooms or factories, not just controlled labs.
Addressing these hurdles keeps the solution affordable for education, rehabilitation, and home entertainment.
Conclusions and Future Directions
The research demonstrated that low-cost webcams and a modern CNN can map real-world objects into VR at interactive frame rates, markedly improving immersion through genuine tactile feedback. However, symmetric shapes, heavy occlusions, and domain shift between synthetic and live imagery remain open problems. Immediate next steps include:
- Incorporating a second (or third) camera for viewpoint diversity.
- Exploring symmetry-aware loss functions and rotational-equivalence labelling.
- Expanding datasets with real-world captures to complement synthetic images.
With these refinements, VR can inch closer to an experience in which what you see is exactly what you touch—no expensive gloves required.
Download and read the full research here: https://scholar.sun.ac.za/items/42b3d715-5cec-4dce-8579-096dc6c23084