South Africa has displayed a unique energy supply profile over recent years, where the ability to meet the energy demand consistently has been constrained by physical limitations of the current energy supply infrastructure. This resulted in countrywide loadshedding events, where total energy supply within high-demand periods cannot be met.
Reasons for the country’s energy supply deficit include:
- Low-grade coal, poorly maintained power plants and the impending decommissioning of existing thermal plants.
- Inadequate supply in high-demand periods typically requires a response from expensive on-demand dispatch units, which are often non-renewable resources. This also equates to a decrease in grid supply stability.
Background to the study
The need for transitioning to a more sustainable energy supply has resulted in a surge in renewable energy investment interest. Wind and PV resources have become the leading green technologies of the renewable energy sector. However, from a grid perspective, these energy sources are non-dispatchable and exhibit a high degree of variability and uncertainty. Optimising spatially allows the variability associated with the combined wind and PV generation fleet to be substantially mitigated.
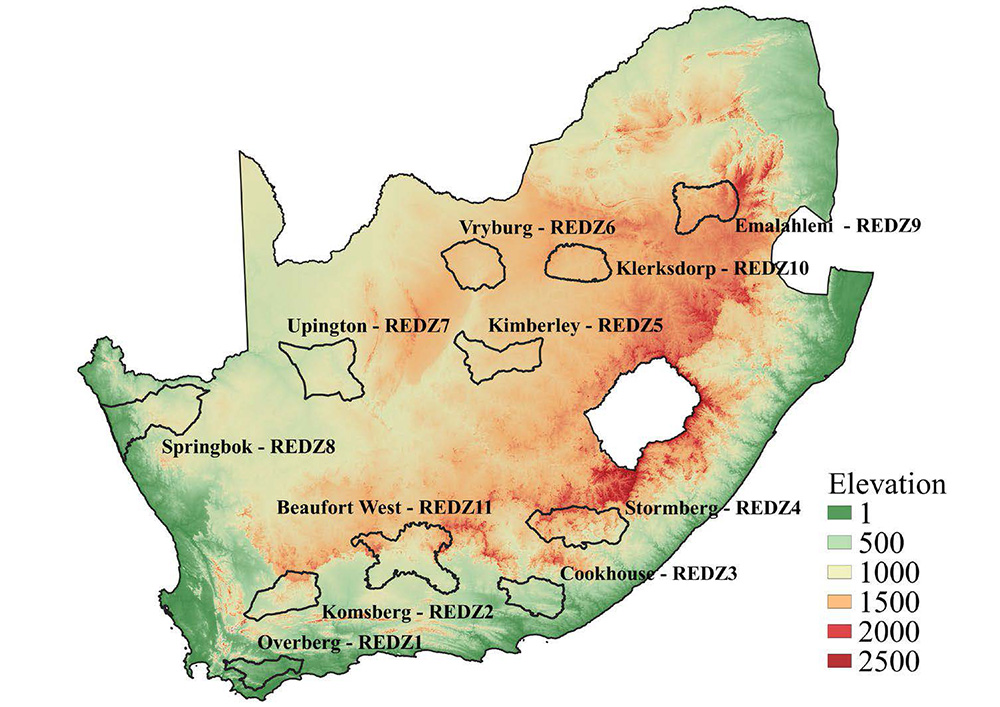
In light of the above, and as part of her doctoral research, Dr Chantelle van Staden developed a renewable energy resource planning framework to be used by government, public electric utility, and private electric utility. She conducted her research under the supervision of Professor Hendrik Vermeulen – they are both from the Electrical and Electronic Engineering Department at Stellenbosch University.
The research approach – renewable energy resource planning framework
In support of a reliable future energy supply scenario with high penetration of renewable energy, the optimisation framework proposed in the research discussed here represents a probabilistic risk-based approach. It seeks to minimise the number of events where high residual load values require ancillary service interventions to maintain power balance.
In this approach, renewable energy resource features are categorised in terms of the statistical properties of the spatiotemporal wind and solar power profiles for a given set of daily and seasonal Time-of-Use periods. In this context, it is recognised that the resource characteristics and grid impact of wind and solar generation profiles can be interpreted with reference to the daily and seasonal cycles exhibited by the demand profiles, wherein some Time-of-Use periods are more important than others.
Apart from the benefit of assigning renewable energy capacities to spatial regions rather than specific coordinates, clustering dramatically reduces input data sets’ dimensions. This reduces the dimensionality of the multi-variable optimisation search space, which translates to reduced local minimal risk and computational cost.
Conclusion
The proposed framework has been implemented for several baseline and optimisation case studies. It is concluded that the framework is highly flexible in the sense that the formulation of the minimum and maximum allocation constraints allows application for real-world scenarios where capacity allocation constraints apply on a regional level.
Overall, the optimisation framework provides a robust method for the geospatial capacity allocation of wind and solar resources. The framework employs a robust way of handling constraint scenarios when considering multiple highly granular resource clusters.
The complete research can be downloaded and read at: https://scholar.sun.ac.za/bitstream/handle/10019.1/124682/vanstaden_geospatial_2022.pdf?sequence=1&isAllowed=y.